Scientists team with business innovators to solve 'big data' bottleneck
In a study that represents a potential cultural shift in how basic science research can be conducted, researchers from Harvard Medical School, Harvard Business School and London Business School have demonstrated that a crowdsourcing platform pioneered in the commercial sector can solve a complex biological problem more quickly than conventional approaches -- and at a fraction of the cost. Partnering with TopCoder, a crowdsourcing platform with a global community of 450,000 algorithm specialists and software developers, researchers identified a program that can analyze vast amounts of data, in this case from the genes and gene mutations that build antibodies and T cell receptors. Since the immune system takes a limited number of genes and recombines them to fight a seemingly infinite number of invaders, predicting these genetic configurations has proven a massive challenge, with few good solutions.
The program identified through this crowdsourcing experiment succeeded with an unprecedented level of accuracy and remarkable speed.
"This is a proof-of-concept demonstration that we can bring people together not only from different schools and different disciplines, but from entirely different economic sectors, to solve problems that are bigger than one person, department or institution," said Eva Guinan, HMS associate professor of radiation oncology at Dana-Farber Cancer Institute and director of the Harvard Catalyst Linkages Program. "Given how complicated the immune system is, this has been a particularly formidable biological problem, and building tools for solving it has been hard and time-consuming. We were stunned by the power of these results and their potential application."
"This study makes us think about greater efficiencies in academic research can be obtained," said Karim Lakhani, associate professor in the Technology and Operations Management Unit at Harvard Business School. "In a traditional setting, a life scientist who needs large volumes of data analyzed will hire a postdoc to create a solution, and it could take well over a year. We're showing that in certain instances, existing platforms and communities might solve these problems better, cheaper and faster."
"We're excited to see that ideas from economics and management fields can be so productively applied to medical research," said Kevin Boudreau, assistant professor of strategy and entrepreneurship at London Business School. "This progress is heartening, particularly in view of the computational challenges we face in understanding so many diseases. We hope this provides a model of how social science and medical researchers can collaborate to solve real-world problems that matter to people."
These findings are reported Feb. 7 in Nature Biotechnology.
For several years Boudreau, Guinan and Lakhani -- through Harvard Catalyst -- have explored the potential applicability of open and distributed innovation approaches to new areas, such as medical research. This has involved bringing insights from social science and economics to processes of medical research. They teamed up with Ramy Arnaout, HMS assistant professor of pathology at Beth Israel Deaconess Medical Center. Arnaout is also a systems biologist whose laboratory studies immune sequencing and other so-called "big-data" problems in biomedicine. Arnaout had developed computational methods for analyzing immune repertoires, but he could foresee having to invest significant computer and personnel resources to keep those methods able to handle the ever-increasing influx of data.
The researchers offered TopCoder what they thought would be an impossible goal: to develop a predictive algorithm that was an order of magnitude better than either Arnaout's or the NIH's standard algorithm (known as BLAST), and that could scale up to the mounting data demands. To do this, they had to first reframe the problem, translating it so that it could be accessible to individuals not trained in computational biology.
In only two weeks, viable solutions came from 122 different individuals. Among these, 16 were more accurate -- and up to 1,000 times faster -- than BLAST. The research team has released the top five performing code submissions under an open source license.
"This is more than just a quick, in expensive answer," said Guinan. "It's uniting different approaches to a problem by taking from Harvard many disparate reservoirs of knowledge and bringing them together to formulate the question, analyze the data, and then put it back to use. This draws on our faculty in a very diverse way. By extending the numbers of people who look at our specific problem, we get solutions rapidly. We have a lot of biases about doing that, and we really shouldn't. In the end this allows researchers to turn their attention to basic science questions and not get caught up in details that they are less well suited to address."
"In a way, the immune system is really the dark matter of biology," said Arnaout. "We have all this sequence data, and there's no good way to figure out what it's doing. Not only did the best entries achieve truly superior performance, but also this kind of crowdsourcing has the potential to be a general solution for a whole class of problems in biology. No single university or institution has the bandwidth and resources to achieve this kind of result so quickly and efficiently."
Co-authors on the study included Po-Ru Loh (Massachusetts Institute of Technology), Lars Backstrom (TopCoder), Carliss Baldwin (HBS), Eric Lonstein (HBS), Mike Lydon (TopCoder) and Alan MacCormack (HBS).
This work was funded by Harvard Business School's Division of Research and Faculty Development, the NASA Tournament Lab at Harvard's Institute for Quantitative Social Science, and Harvard Catalyst.
Source: Harvard Medical School
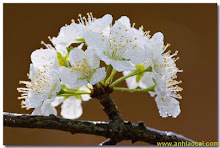